HadCRUT4: Analysis and critique
Posted on 13 June 2012 by Kevin C
In my previous three articles on HadSST3, CRUTEM4 and HadCRUT4, I have given an overview of the literature and data concerning the new datasets which comprise the Hadley/CRU version of the instrumental temperature record. The analysis I have presented so far has been addressed at communicating the work done by Hadley and CRU as clearly as possible.
However in the course of examining the data for these articles I have come across a number of features which are of interest in understanding the data and do not seem to have been widely reported. Some of these features are (at least to me) rather unexpected. Note however that this material is the result of a few months of spare-time effort, and has not been subject to the scrutiny of peer-review, and so should be treated as tentative. It is likely that at least some of it is wrong. Constructive criticism and pointers to any previous similar work I have missed are welcome.
The material is quite dense. Much of it concerns the problem of coverage bias, so reviewing my previous articles ‘HadCRUT3, Cool or Uncool?’ and ‘GISTEMP, Cool or Uncool?’ on this subject may be helpful. I will start by presenting an outline of my conclusions and then explain in detail how I reached them.
HadCRUT4: Surprises and a coincidence
For me the HadCRUT4 dataset contained two big surprises:
-
The first surprise concerned coverage bias. Studies from the ECMWF and GISS have both identified significant coverage problems in the HadCRUT3 dataset, leading to a significant underestimation of recent temperature trends. I expected the HadCRUT4 update to focus primarily on addressing this. However the improvement in global coverage is actually rather small. Instead the Hadley/CRU collaboration found some different biases and addressed those.
-
The second surprise concerns the year 1998. Multiple factors have been conspiring to inflate temperatures around 1998 and/or suppress trends starting in the mid 90's or later. In addition to the 1998 El-Nino, these factors include poor coverage of the Arctic, poor coverage of the Antarctic and a discontinuity in the old HadSST2 data. HadCRUT4 does resolve the SST discontinuity and part of the Arctic coverage bias, but not the Antarctic coverage bias.
In the light of this it becomes clear why we see so many trends starting around 1998: Three different biases have contrived to cause the temperatures around that year to be overestimated over and above record-breaking El-Nino level, and two of these biases also supress trends starting in or after that year.
Background
In order to understand the impact of incomplete coverage on a global temperature estimate, we need to know how temperatures have varied over different regions of the globe. This figure from Columbia University shows temperature anomalies in 5 latitude bands:
Figure 1: Temperature anomalies with latitude band.
Note that apart from a period around the second world war, temperatures have varied consistently across the globe from about 1920 to 1980 (indicated by the lines running parallel - the fact they are grouped is an artifact of the anomaly calculation). However after 1990 the temperature anomalies for different latitude bands show very different behaviour. Different parts of the planet respond at different rates to rapid greenhouse forcing. This will be important in understanding some of the results presented below.
HadSST3 analysis
One of the most interesting parts of this paper to me was the following quotation:
"It should be noted that the adjustments presented here and their uncertainties represent a first attempt to produce an SST data set that has been homogenized from 1850 to 2006. Therefore, the uncertainties ought to be considered incomplete until other independent attempts have been made to assess the biases and their uncertainties using different approaches to those described here."
I read that as follows: “Here’s a problem, it’s important, but it’s also hard. We’ve given it our best shot, but can’t be sure we got it right. Other groups need to tackle the problem and challenge our results to sort this out properly.”
In my view that is scientific skepticism at its finest. When addressing a new problem it is common for early work to be flawed. It usually takes several cycles of fault-finding before the problems are ironed out and a consensus begins to emerge. But this is seldom so clearly acknowledged, and it is not uncommon for the authors of an early work to be the last to accept that they may not have got it right first time.
How might the HadSST3 work be challenged? We have seen that the HadSST3 adjustments are fairly simply related to the mix of observation types and the biases in those observations. As a result to obtain a different result from the same source data would require either:
-
A significant change in the estimated magnitudes of the biases associated with any measurement type (which may in turn may vary with time or measurement type).
-
A significant change in the observation classifications, resulting in a change in the time evolution of the mix of observations.
Since the SST biases potentially impact the GISTEMP and NCDC records it will be interesting to see how other groups respond to this work, and whether their conclusions are similar.
There is one other feature of HadSST3 which I have not covered until now. In HadSST3: A detailed look I showed this graph of the difference between HadSST3 and HadSST2, compared to the change in adjustments. Note there has been a significant downward correction to HadSST3 starting in 1998, excerpted in the left hand panel of Figure 2. The difference is not due to the HadSST3 adjustments.
Figure 2: 1998 discontinuity in the HadSST2 data.
A comparison of HadSST2 with the NCDC temperature data, and of the unadjusted HadSST3 (i.e. without the new bias corrections) with NCDC using only common coverage ocean cells is shown in right panel of Figure 2. (Some contamination from land stations in coastal cells is also present). This comparison suggests that the problem is in HadSST2, which is overestimating temperatures starting from 1998. There is also support for this conclusion in the metadata; HadSST2 switches from the ICOADS data to the NCEP near-real time product at the beginning of 1998. The data and metadata both suggest that the discontinuity is in the HadSST2 dataset.
The impact of this discontinuity is an overestimation of SSTs starting in 1998. There is weak evidence of a subsequent decline, which would cause trends starting after 1998 to be underestimated.
CRUTEM4 analysis
In CRUTEM4: A detailed look, I pointed out the difficulties in providing a comparison of the CRUTEM4 data with the other land-only temperature datasets from NCDC, GISS or BEST due to problems created by different definitions of ‘land-only’, and different averaging and baseline conventions. Here is my best effort at such a comparison.
I infer from their methods paper that BEST team have produced a land-masked global average; which I described as method 1A. I therefore applied this method to the gridded CRU and GISS datasets to produce a like-for-like comparison. I have included both the GISS land Temperature station (dTs) datasets - dTS 1200km and dTs 250km - which differ in how far temperature data may be extrapolated from a weather station. The results over two different timescales are shown in Figure 3 using a 60-month moving average (click the controls to see the second graph).
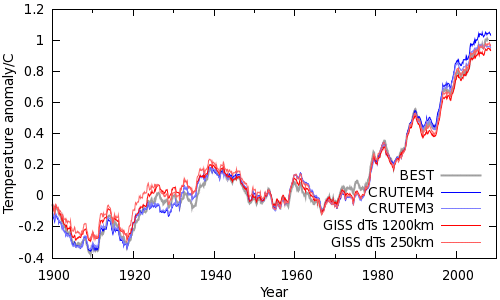
Note that agreement is fairly good between the BEST and GISTEMP data. CRUTEM3 overestimates temperatures around 1998/9, then falls back into line with the others. CRUTEM4 is similarly warm around 1998, but stays warm to the present.
If this is correct then CRUTEM3 underestimates recent trends as much because it was warm at the beginning of the trend period as because it is cool now. CRUTEM4 produces more realistic short term trends, but only because it is consistently warm.
Those of you who remember the first comparison graph released by the BEST project may be startled by this result, which is why I didn’t report it earlier. (You might want to check the current version, which is rather different.) The earlier BEST graph has understandably been interpreted as evidence that CRUTEM3 is an outlier (for example by Dana here and here). Figure 3 leads to the same conclusion, but for different reasons.
What is going on? It all comes down to coverage bias. We can estimate the bias in the CRUTEM3/4 data due to poor coverage by using another dataset with good coverage; in this case the GISTEMP land-only product, GISS dTs 1200km, which has 100% coverage (as opposed to CRUTEM3 - 60% and CRUTEM4 - 70%). We can mask the gridded temperature map for each month of the GISS data to reduce its coverage to match the CRU data for the corresponding month. If we calculate temperature series for both the masked and the complete data, and take the difference, the result is the bias which would be introduced into the GISS data if its coverage were no better than CRU. This may be used as an estimate of the bias in the CRU data due to poor coverage. This result is independent of the global temperature for the GISS data, it depends only on how those temperatures are distributed between areas which are present or missing from the corresponding CRU map for that month.
The results are shown in Figure 4 (60 month moving average). (Note: For strict validity the anomaly baseline period of the GISTEMP map series was first adjusted to match the CRU data.)
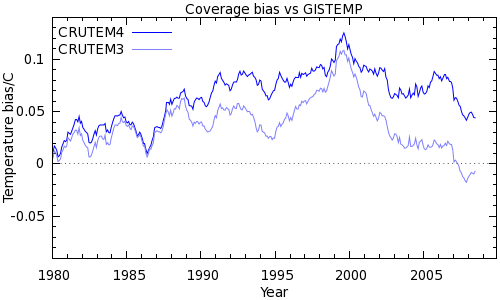
To be totally clear, this is not a difference between GISTEMP and CRUTEM; it is a difference between GISTEMP (reduced coverage) and GISTEMP (full coverage).
The bias calculation confirms that around 1999 CRUTEM3 should, on the basis of coverage alone, be biased warm, however that bias should decline rapidly after that point. CRUTEM4 is also biased warm, but the bias persists to the present day with only a smaller decline.
Just to make sure, I repeated the same calculation using the UAH satellite data. This is not a fair comparison, since lower troposphere (LT) temperatures and surface temperatures behave differently, but it is a completely independent test. The results are shown in the second part of Figure 4 (click the 'from UAH button'), and show the same pattern but with a rather muted signal - this is because the geographical variation in LT temperature anomalies is rather smaller than for surface temperatures. (As with GISTEMP the anomaly periods must match, so both CRU and UAH map anomalies were baselines with respect to 1981-1990.)
Both GISTEMP and UAH suggest that the differences we see in Figure 3 are due to coverage bias. How does this arise? This can be answered by breaking down the coverage bias contributions into latitude bands, shown in Figure 5. (Two plots for CRUTEM3 and CRUTEM4 respectively, 60 month moving average.)
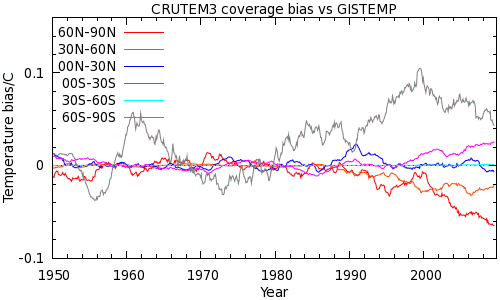
The big contributor to the warm bias around 1999 is the Antarctic, which is barely covered in the CRUTEM3/4 data. The decline to a cool bias in CRUTEM3 arises both from the Antarctic and from the high Northern latitudes. Note that, excepting the Antarctic, the lines remain well grouped until about 1990, when they start to scatter, just like the zonal anomalies in Figure 1.
In CRUTEM4, the Antarctic bias is very similar, but improvements in coverage have reduced Northern high- and mid-latitude bias. The lines are still diverging however, so careful monitoring for future bias will be required.
Why does the missing Antarctic cause a warm bias? From Figure 1 we see that Antarctic temperature anomalies have declined slightly since 1970 (presumably due to the ozone hole) while the rest of the planet has been warming. Omitting a cooling region imposes a warm bias. As the Antarctic starts to warm after 2000, the bias reduces.
The underlying issue is this: While the planet was subject primarily to natural changes, the different parts of the planet were warming and cooling at similar rates, thus the zonal anomalies run fairly parallel. As a result, poor coverage does not create significant bias. Under rapid greenhouse warming, the different parts of the planet have warmed at very different rates, leading to the scatter in the zonal anomalies since 1980. At this point poor coverage becomes a very significant source of bias. To track changes in global temperature, we need much better coverage now than we did in 1980.
HadCRUT4
One interesting feature of the HadCRUT4 paper is the use of the NCEP/NCAR reanalysis data to obtain an estimate for the coverage uncertainty. The approach is very similar to the one I adopted using the GISS dTs data above, with one important difference - every month in the reanalysis data is used to determine a distribution of possible coverage biases for each individual month in the HadCRUT4 record.
But, as we’ve already seen, the impact of coverage has changed significantly over recent decades. Using the whole period of data leads to an uncertainty estimate which is a compound of the desired uncertainty, and a bias estimate based on an average over the total span of the reanalysis dataset. A better approach would be to use a window of months about the current month to obtain a time-dependent estimate of both the bias and the uncertainty due lack of coverage. Separating out the bias term will in turn reduce the uncertainty. From Figure 1 it looks as though a window of no more than 120 months, and preferably only 60 months is desirable to capture the changing distribution of temperature anomalies, however a shorter window may not provide enough data to reliably estimate the uncertainty.
We can apply my simpler bias analysis (which we can now see is limited in that it does not provide an uncertainty estimate for the estimated bias) to HadCRUT3/4. Coverage bias estimates are shown for both HadCRUT versions using the GISTEMP land-ocean series and the UAH series to provide the temperature maps. The results are shown in Figure 6 with a 60 month moving average.
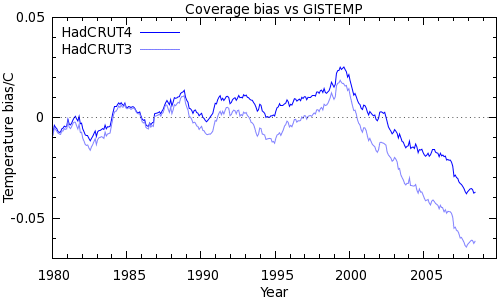
See also zonal coverage plots for HadCRUT3 and HadCRUT4.
The impact of coverage bias on recent trends has been reduced, but only slightly. Looking at the zonal plots, most but not all of of the Arctic bias has been dealt with, but the Antarctic bias is almost unchanged, despite the introduction of Antarctic stations in HadCRUT4 (the equal angle grid used by CRU means that these stations cover a tiny area). Both series show a weak warm bias around 1998 followed by a rapid transition to a strong cool bias driven by the missing data from both poles. The additional high latitude data in CRUTEM4 (and to a lesser extent HadSST3) only partially mitigates this trend.
The UAH data shows the same pattern, although again the effect is muted due to the reduced geographical variation of the lower troposphere data.
What does this mean for the temperature trends? HadCRUT4 now gives rise to trends since 1998 which are much more in line with GISTEMP. This is despite the coverage bias being largely unaddressed. The apparent convergence in short term trends between HadCRUT4 and GISTEMP arises primarily from changes in the HadSST data over areas which already had coverage, not through addressing the global coverage issues.
The following table gives the 15 year trends over the period 1996-2010 for GISTEMP, NCDC, HadCRUT3, and HadCRUT4:
Dataset | Trend | Coverage | Common trend | Common coverage |
GISTEMP | 0.157 | 100% | 0.089 | 79% |
NCDC | 0.121 | 91% | 0.095 | 79% |
HadCRUT3 | 0.098 | 80% | 0.098 | 79% |
HadCRUT4 | 0.136 | 84% | 0.119 | 79% |
Looking at the trends in the first column, the HadCRUT4 update seems to have brought the Hadley/CRU dataset into better agreement with GISTEMP and NCDC (Dana commented on this in his ‘first look’ post). But also look at the coverage figures in the second column: For GISTEMP, NCDC and HadCRUT3, the reported trend declines very significantly with declining coverage. Globally HadCRUT4 only provides a marginal improvement in coverage, but the trend leaps up to fall between GISTEMP and NCDC.
What is going on? The clue is in the third column of the table, which shows the trend calculated only over the map cells where the datasets all share common coverage. GISTEMP, NCDC and HadCRUT3 all show good agreement, and HadCRUT4 is the outlier. Why? Because GISTEMP, NCDC and HadCRUT3 are all using current generation SST datasets, and HadCRUT4 is using a next generation SST product with additional bias corrections. This can also be seen in the final figure from Morice et al 2012 (detail here), in which HadCRUT4 is at the top of the pack over recent years, although GISTEMP and NCDC both lie within the uncertainty range.
In other words, the apparent agreement in trends is potentially misleading. HadCRUT4 still has significant coverage bias (confirming the result from Figure 6) which is somewhat masked by the increase in SST trend.
The HadCRUT3 1998 anomaly
The super El-Nino of 1998 and its impact on temperature trends is well known. Less well known is that HadCRUT3 appears to have suffered from multiple biases all of which served to inflate temperatures around 1998, and thus create a spurious cooling trend since that date. The biases due to poor coverage in both the Arctic and Antarctic peak in 1998 for HadCRUT3. As we have seen the Arctic bias is significantly improved in HadCRUT4 however the Antarctic bias remains.
The additional bias due to the HadSST2 discontinuity may be estimated from the difference between HadSST2 and HadSST3 with the new adjustments removed (at least until 2006 when the published data ends). The combined coverage and discontinuity biases are shown in Figure 7. A 12-month (rather than 60 month) moving average has been used in this case, revealing the substantial year-on-year variation in the coverage bias.
Figure 7: HadCRUT3 1998 biases.
The trend lines quantify the impact of the biases on the HadCRUT3 trend, and have slopes of between 0.09 and 0.11°C/decade. It looks as though most of the difference between the recent and long-term trends in HadCRUT3 can be explained by just the coverage bias and the impact of the El-Nino cycle.
Discussion
One problem in my own field is that we are involved in producing data which is consumed by users with very different expertise from our own. Sources of bias and/or uncertainty which are obvious to us may not be obvious to people who make use of the data we produce. The problem of communicating the results, uncertainties, and limitations of a dataset are challenging.
You don't always get to pick your users. In climate science this has become an issue because the media and the public have become users of the instrumental temperature record data without necessarily understanding it. It has been adopted, rightly or wrongly, as a metric for the correctness of climate science. Issues of coverage are likely to be lost on the man in the street, who will therefore have no grounds for evaluating different temperature records. Commentators with an agenda to pursue will select the dataset which best conforms to their agenda.
I am not competent to judge the correctness of the HadSST3 bias corrections: That is a discussion which will take place in the peer-reviewed literature over the next few years. However, the impact of coverage bias is pretty clear; it can be seen by simply looking at a coverage and anomaly map as we did here, or by assessment of coverage bias using GISTEMP, or by the less valid but independent assessment using UAH. Coverage bias was less of an issue before 1980 because there was little difference in trends between latitude zones. It has become an issue with the rapid and geographically stratified warming of the past 30 years, and if current trends continue it will become more of an issue in future.
If the major records go on reporting divergent results which are not representative of the global surface temperature, then it seems likely that this will continue to be a source of confusion to the public. I would therefore argue that, while the gridded datasets remain of vital interest to specialist users, it is incumbent on the major records to report some kind of unbiased estimate of global mean temperature for public consumption.
The change in climate norms over recent decades makes this a non-trivial problem, and it may be that different methods are required for recent and long term records. Alternatively the Kriging approach adopted by the BEST project seems to me to be a promising tool for achieving near complete coverage over recent years, while degrading gracefully as we go back in time. If so, a BEST land-ocean product may change the situation.
In the meantime I hope to offer my own rudimentary solutions to the problem of coverage bias in a forthcoming article.
I've noticed the MET stopped issuing their climate bulletins at the end of 2014, and the HadCrut4 data seems to end in March 2015. Yet ver. 4.4.0.0 was released, looks like in August. Is there a disconnect somewhere?
knaugle @3, this page for monthly data. This page for links to all HadCRUT4 data. Data format described here. The data is currently available through to August, 2015.